CS Student Posters and Talk at Mount Sinai Undergraduate Research Symposium
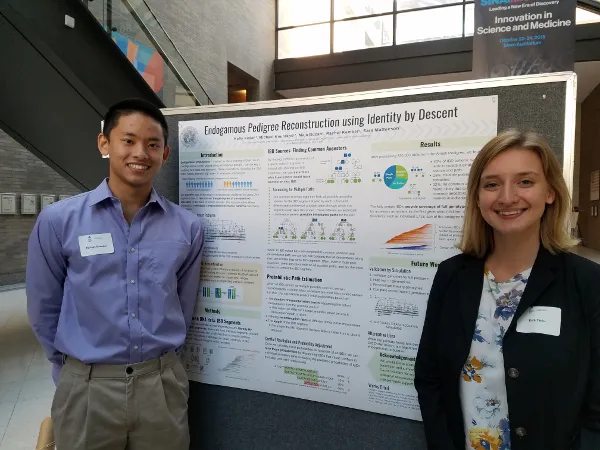
Swarthmore Computer Science students Kelly Finke '21, Michael Kourakos '21, and Nhung Hoang '19 presented their research at the Mount Sinai Undergraduate Research Symposium in New York City this month. They all worked on this research over the summer (2018) with Visiting Assistant Professor Sara Mathieson.
Kelly and Michael presented their work on Ancestral Genotype Reconstruction (see below) as a poster. Their project was selected for a talk which was given by Kelly. Nhung presented her research (joint work with Hunter Lee '19) on using machine learning to decipher population histories (also see below) as a poster and won a Best Poster award.
Ancestral Genotype Reconstruction in Endogamous Pedigrees
Kelly Finke and Michael Kourakos
As DNA sequencing decreases in cost, genetic studies of individuals andfamilies becomes increasingly accessible for biologists investigatingdisease inheritance; still, however, it is often impossible to obtaingenetic information for an entire family tree, especially if that pedigreeextends many generations back in time. Since it is often useful forbiologists to examine complete, multigenerational pedigrees, a number ofalgorithms attempt to fill these gaps by using known genotypes of livingindividuals to construct estimations of unknown genotypes of recentancestors. However, many of these algorithms fail when working withcomplicated pedigrees, such as pedigrees that involve inbreeding,cross-generational marriages, or remarriage. When working with endogamouspedigrees - pedigrees marked by a close sharing of DNA due to the practiceof marrying within a small, contained group - all of these complicationsare common. Our endogamous pedigree reconstruction algorithm, therefore, isdesigned to take these factors into account, allowing users to work bothwith typical pedigrees and more complex, endogamous pedigrees. Byprobabilistically analyzing the most likely sources of DNA segments sharedbetween related individuals (identity by descent segments), our algorithmis able to reconstruct ancestral genotypes for even very complicatedpedigrees. This algorithm allows biologists to better gain insights fromthese genetically unique populations which can inform studies of raretraits and diseases that are disproportionately expressed in endogamouscommunities.
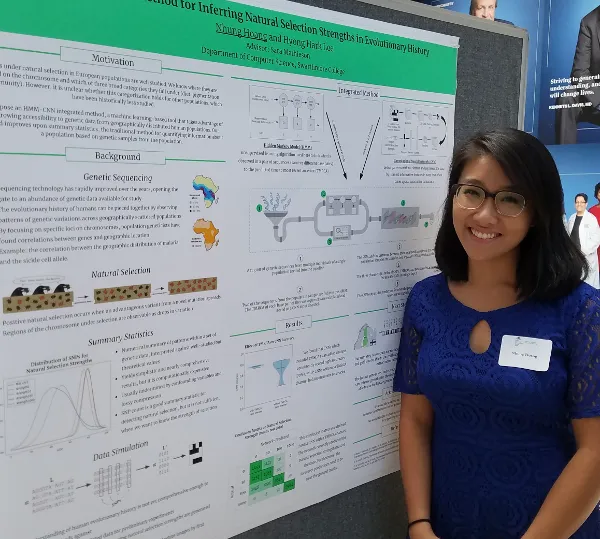
An HMM-CNN Method for Inferring Natural Selection Strengths in Evolutionary History
Nhung Hoang and Hunter Lee
The evolutionary histories of human populations are traditionally studiedand inferred using summary statistics. This quantitative approach yieldssimplistic and nearly comprehensive results, but it is computationallyexpensive and usually undermined by confounding variables, as well as lossycompression. Recent work in population genetics has turned to machinelearning to take advantage of the current abundance of genetic dataavailable. We propose a Hidden Markov Model (HMM) to Convolutional NeuralNetwork (CNN) pipeline that improves upon the summary statistics approach.
HMMs are unsupervised statistical models that are effective at generalizingglobal trends across the genome. CNNs, a class of machine learning models,are trained to extract local patterns within a given set of data and torepresent the data by its most informative features. Our objective is tointegrate the advantages of the two models into one pipeline for makingevolutionary inferences about populations. Specifically, our integratedmethod is designed to take in samples of genetic regions from a populationand produce a prediction for how strongly natural selection has affectedthe population in that genetic region. Our results, for population datagenerated using coalescent simulators, show that the global informationlearned by the HMM helps the CNN in capturing local information about thegenetic data, thus producing consistently high accuracies for inferringnatural selection strengths.