Jordan Ellenberg: Arnold Dresden Lecture 2015
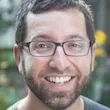
Math is thought of as a discipline where the point is to get the right answers. In fact, the interesting part of math is much more likely to concern asking the right questions. And when it does come time for answers, math is not black and white; it concerns itself with uncertainty and contradiction. In this lecture, Jordan Ellenberg tells some math stories concerning World War II bombers, Nate Silver, a summer job he had in college, and the Pythagorean theorem; recites some poetry; argues against Theodore Roosevelt; and tries to make the case that thinking like a mathematician is especially useful in domains of uncertainty, ambiguity, and apparent paradox.
Jordan Ellenberg is the John D. MacArthur Professor of Mathematics at University of Wisconsin. Ellenberg regularly contributes to Slate and has written articles on mathematical topics for The New York Times, The Washington Post, The Boston Globe, and Wired. He is the author of the best-selling book How Not to Be Wrong. Ellenberg spoke on campus as part of the 2014-15 Arnold Dresden Memorial Lectures, named for an esteemed mathematics professor who taught at Swarthmore from 1927-1952.
Audio Transcript
Jordan Ellenberg: Let me just start by telling you guys about my summer job that I had when I was in college. I was a math major, so I didn't get a normal summer job. I got a math summer job, and the job was that there was a biological researcher on campus who wanted to know how many people were going to have tuberculosis in the year 2050, which, actually, when I was in college, seemed like a long time away. Now it doesn't seem so ... That was an exciting problem, and this is exactly the kind of thing that mathematical biologists do, so I xeroxed a whole bunch of papers about the spread of tuberculosis, about its etiology, about its relative frequency in different populations, and what you can do with all this stuff is you can build a model.
This many people in this country are infected with the disease. This many people are contagious. You make little differential equations for how it varies from year to year, how these things interact with each other, and you set up your model and you let it run. You let it run year after year. You iterate it, and you see what happens. I did that and I got an answer and I gave it to my boss and then it was the end of the summer. That was my summer job. That was the end of the summer job, but it wasn't really the end of the story, because I thought about this a little bit more as summer turned to fall. Of course, every paper you read in empirical biology will give you a point estimate for some constant, like the rate of contagion of a certain disease, but that estimate has error bars around it. If it says something happens 13% of the time, what does that mean? It means, might be 10% under some condition, might be 18%, probably not 1%, probably not 80% under any conditions, but there's a range.
If you put that into your model and you say, "What if it wasn't exactly what I thought it was, but it was a little bit different," and there's a lot of little pieces of this model and they all feed back into each other. As you iterate year after year, what happens is that these uncertainties start to feed back into each other and they start to multiply until actually, before too long, the noise completely engulfs the signal. What I learned, when I undertook this exercise, was that, in point of fact, I actually had no idea how many people were going to have tuberculosis in the year 2050. This was my new answer to this question: As far as I knew, the disease could be completely extinct or the disease could be completely endemic throughout the entire world.
I went back to my boss, actually, and I was like, "Look, I know I had this job and you paid me and everything, but actually I think neither you nor I has any idea how many people are going to have tuberculosis in the year 2050." It was a life lesson for me because it turned out that my boss actually didn't really care about this and did not want to hear what I had to say. He was perfectly satisfied with my deliverable on this job. To be honest, what I find sort of pointed and sad about that story is that I'm sure that that guy went around giving a million talks where he said how many people are going to have, projected to have, tuberculosis in the year 2050, and if somebody asked him how he knew that, he would say, "Well, I hired a guy and he did the math."
All right. I started with this sad story to get us all into a somber mood, but let me tell you another story. I'm just going to tell a series of stories about uncertainty that link together. Here's another one with a bit of a happier ending.
Here's a mathematician. This is Abraham Wald. Who is he? He's a guy, who actually starts out studying pure math, studying super abstract point-set topology. He's in Austria. When the Nazis take over, he has to leave, as a lot of people do, and when the smoke clears, he's in Columbia. He's a professor there. He manages to get to the United States. During World War II, he's working at an institution called the Statistical Research Group. It's actually not so well known, but it was this top secret installation of some of really the strongest mathematicians and statisticians of the time doing secret work related to the war, all in this apartment building in Morningside Heights right by Columbia. It was kind of like The Manhattan Project, except it was actually in Manhattan.
One day ... I was looking at my slide, but I assume everything's okay back there. One day, a group of generals came to the Statistical Research Group, they came to the SRG and to Abraham Wald with a question. They were looking at the planes that came back from flying missions over Germany. When a plane comes back from a mission, it looks kind of like this, riddled with bullet holes, right? What they had noticed is the bullet holes were not evenly distributed across the plane. It wasn't a uniform distribution. In fact, I'll show you a little table.
For instance, there were more bullet holes on the fuselage and fewer on the engine, for example. The table's more complicated than this, but this is what they were saying. What they came to ask was, "Where should we put the armor?" This is a real issue if you're designing a fighter plane, because if you put too much armor on it, the plane can't fly. Okay, that's bad. That doesn't work. If you put too little armor on it, the plane gets shot down, which is also bad. There's clearly some kind of trade-off here, some kind of optimization problem.
What they wanted to know is: We can see that the planes are getting hit more on the fuselage than they are on the engine, more on some parts than others. How much more armor should we put in those places where the plane is getting hit more? Is there some kind of a mathematical formula or an equation so that you can tell us the exactly optimal thing to do? That was the question that the generals brought to the mathematicians.
Wald gave them an answer, but it was not the answer they were expecting at all. I see all the smiling people are people who read my book, right? Is that right? Wald gave them an answer. He said, "No. You're completely wrong. You have to put the armor where the bullet holes are not. You have to armor the engine and leave the fuselage alone." He let that sit for a second. What's going on? "What's going on," Wald explained, "is that it's not that the Nazis can't hit your planes on the engine. It's that the planes that got hit on the engine are not the ones that are coming back from the missions."
This seems to me to be an absolutely classic piece of applied mathematics that shows exactly what mathematicians are put on this earth to do. There's sort of a stereotype I think that, probably not ... I mean, a lot of you guys are from the department, so you, I'm sure, don't have this stereotype, but maybe your friends do. The mathematician is like a super advanced human calculator, like a sort of Texas Instrument on two feet, that what we are here to do is to give numerical answers to questions, and that's certainly what the generals came to Wald asking for, but that is not really what ... It's one of the things that mathematicians are, but it's not the sum total.
What we are really good at is actually formulating the right questions and interrogating a question given to us to figure out whether it's really getting at the issue, the issue that's important, and, in this case, it was not. In this case, the generals were coming to them not quite with the right question. When I think of this story, sort of bear with me here, I think of ... Everybody know who this is? Who's going to identify this great hero of American history? Who is it?
Audience: Teddy Roosevelt.
Jordan Ellenberg: It's Teddy Roosevelt, in his dashing Cuban days. It's Teddy Roosevelt, and he has a very famous speech that I always think about when I hear this story of Abraham Wald. I'm going to read a piece of it. It starts out ... It's a speech called Citizenship in the Republic. He starts out, "Strange and impressive associations arise in the mind of a man who speaks before this august body in this ancient institution of learning." I like that. It's not Swarthmore he's talking about. He's at the Sorbonne. He's in Paris, talking at the Sorbonne. It's 1910, so he's just finished being President. The speech is very long. It's actually very interesting. I highly recommend reading it, and you'll cry when you think of the kinds of speeches Presidents give today, by comparison, but anyway I'm going to read the very famous part of this speech, which many of you have probably heard.
He says, "It is not the critic who counts, not the man who points out how the strong man stumbles or where the doer of deeds could have done them better. The credit belongs to the man who was actually in the arena, whose face is marred by dust and sweat and blood, who strives valiantly, who errs, who comes short again and again, who knows the great enthusiasms, the great devotions, and spends himself in a worthy cause, who, at the best knows in the end the triumphs of high achievement, and who, at the worst, if he fails, at least fails while daring greatly, so that his place will never be with those cold and timid souls, who know neither victory nor defeat."
Who knows it, by the way? Is this a familiar speech to some of you guys? It comes back again and again. Franklin Delano Roosevelt, Theodore's cousin, uses this speech in his last reelection campaign in 1936. Richard Nixon quotes it in his resignation speech, which I like to watch every year on the anniversary in August. Brene Brown has a very popular self-help book, called Daring Greatly. Her TED talk on this was watched four million times. It is very popular as a way to rev yourself up. I want to push back on it a little bit and say, "What's the problem with it?" because when I hear this speech, when Teddy Roosevelt sneers at the cold and timid souls, who sit on the sidelines and second-guess the real warriors, I think about Abraham Wald.
This is a guy, who, as far as we know, never lifted a weapon in anger, but he played a serious part in the American war effort, making these recommendations that were used, not just in World War II, but further in Korea and Vietnam. It became a standard part of Air Force terminology. How did he fight the war? He did it exactly by counseling the doers of deeds how to do them better. He was unsweaty, undusty, and unbloody, but he was right, and he was, in both sense of the words, a critic who counts. You see what I did there, good, okay.
Let me turn to another heroic dude. Who knows who this is? This is harder. Who is it?
Audience: Nate Silver.
Jordan Ellenberg: It's Nate Silver. I love this guy. I hate to admit it, because I'm very proud of my profession, but this is a man who taught more math to more Americans in 2012 than all the math professors in the country put together. I wish it weren't so, but it is. What made him so good?
He's a guy, as some of you know, who specializes in doing quantitative analyses of elections, especially elections that have not happened yet, which are the ones that people are really interested in. Let me just give a sense of why Nate Silver is so good, remind you of what political punditry usually looks like when Nate Silver is not a guest on the program. Okay, so it looks ... This is a little bit out of date, but he becomes famous in 2012, so let me show you. Remember that guy?
Okay, anyway, this is how it works. There'll be a host, and the host will have a Republican guest and a Democratic guest, and they'll say, "Republican Guest, who's going to win the election, Romney or Obama?"
The Republican guest says, "Oh, Mitt Romney's definitely going to win. Here's three reasons: Reason one, reason two, reason three. Bang!"
Okay, "Over to you, Democratic Guest. Who's going to win this election?"
"Ah, Barack Obama's definitely going to win. Here's three reasons: Reason one, reason two, reason three."
Then they do it again the next week. That's what happens. When Nate Silver's on, it looks a little different. They say, "Nate Silver, who's going to win this election?" He gives them something like this. What is this? This is a picture of a probability distribution. This is what Nate Silver would say is the kind of answer that is appropriate when asked, who is going to win the election? This, I think, comes from about a month before the 2012 presidential election.
He would say, "Well, Obama might win and Romney might win, and I think there's different outcomes that are possible, different numbers of electoral votes, and they have different probabilities, according to my best estimate, and I'll make you a little chart showing which outcomes I think are the most likely. Those are the ones of high spikes, and you can see there's a lot more blue than there is red on this picture. The blue outcomes are those where Obama wins the presidency. The red outcomes are those where Romney wins the presidency." This is a picture of Nate Silvers state of knowledge about the election in October 2012, indicating that either guy might win, but that Obama was substantially more likely to win.
I've got to tell you, people hate this. Okay, no, not real people; real people love it. Nate Silver drives a tremendous amount of traffic to the New York Times, where he's working, but the regular political pundits hate it, right? They're very angry that this is considered acceptable. I have some juicy quotes I have to read.
This is Dylan Byers, writing in Politico, which is a major Washington D.C. political magazine. He says of Silver, "This may shock the coffee-drinking NPR types of Seattle, San Francisco, and Madison," so I was very excited because I do drink a lot, a lot of coffee, and I live in Madison and listen to NPR, so I was ready to be shocked, "but more than a few pundits and reporters, including some of his colleagues, believe Silver is highly overrated." He goes on to explain, "So should Mitt Romney win on November 6th, it is difficult to see how people can continue to put faith in the predictions of someone, who has never given that candidate anything higher than a 41% chance of winning way back on June 2nd, and one week from the election gives him a one in four chance. For all the confidence Silver puts in his predictions, he often gives the impression of hedging." This is the quote, okay.
This is the kind of thing I have to tell you, that for people in math it really makes the red haze of rage descend over your vision, because somehow, in other domains, in other domains where uncertainty is present, we accept that it's okay to talk about uncertainty. If we want to know whether it's going to rain tomorrow, and we look on the Internet and look at weather.com or whatever, or we watch the local news and see what the meteorologist says, if they say there's a 40% chance of rain, we do not say, "Why are you hedging? Why don't you just tell us? Is it going to rain or not?" By the way, if they do say it's a 40% chance of rain and then it doesn't rain, we don't say, "I've completely lost faith in that guy. He said there was only a 40% chance of rain. How can I have faith in him ever again?"
This is not the way we treat it, because we understand that things like the weather are subject to uncertainty, and the right answer to the question--is it going to rain or not?--is not yes or no. It's an expression of uncertainty, the uncertainty that we know is there. Maybe the best answer is some attempt to give some kind of quantitative or precise expression to that uncertainty.
Yet, when it comes to things like politics, things having to do with aggregate human behavior, which has got to be a lot more complicated to model than the weather, there suddenly we expect people to give yes or no answers. I think it's a serious problem. Well, we know what happens in the end in the 2012 election. Obama does win the election. Not only that, actually for each state, Nate Silver has a probability model that says the state is more than 50% likely to go to Obama or more than 50% likely to go to Romney, and, in fact, every one of those states falls the way that Nate Silver predicts, and Nate Silver becomes an Internet hero at that time. I wish I made this, but I did not. I just found it. I just found it somewhere.
Then I'm going to tell you that all the existing political class is like, "Wow! Now I'm on board." No, of course not! They hate him even more. I'll read you ... I have another juicy quote, which I think has a lot ... I'm reading this quote, not to pick on people, but because I think, for those of us who are in mathematics and especially those of us who are in parts of mathematics that actually touch the extra-mathematical world, there are certain attitudes out in the culture that I think we have to grapple with. We can't ignore them. We have to grapple them and, to some extent, combat them.
Okay, so here's Leon Wieseltier, writing in the New Republic. Here's what he says about Nate Silver in a long, very strident article. He says, "There is no numerical answer to the question of whether men should be allowed to marry men and the question of whether the government should help the weak and the question of whether we should intervene against genocide, and so the intimidation by quantification, practiced by Silver and the other data mullahs, must be resisted. Nate Silver has made a success out of an escape into diffidence. What is it about conviction that frightens these people?" These people, that's us, folks, these people, these mathy people.
It's amazing the levels of heightened rhetoric that this kind of stuff inspires. I mean, a mullah ... Who could look less like a mullah? I guess, in this kind of rhetoric that is, not just Leon Wieseltier, but it's rather popular. You could think of it as kind of a one drop rule, where there's a sense that if there's a little bit of quantitation, like one formula, one spreadsheet, one diagram, one pie chart, that just that little bit of math turns us all into robots, right? That means we're giving up on our humanity and outsourcing all of our thinking to a calculator.
Those of us who do math know that it's not like that. Nate Silver, by the way, certainly knows that he [inaudible 00:19:41] his beautiful book, The Signal and the Noise. I think it's a brief for humans and computers working together, never one without the other. What I hear in Leon Wieseltier is, in some sense, an echo of Theodore Roosevelt, this sense that what's important is to have conviction. What's important is to have a cause. What's important is to go to the mat and win or lose and not to stand on the sidelines and say, "Maybe it's this. Maybe it's that."
I think Theodore Roosevelt was wrong about that, and I think Leon Wieseltier is wrong about that, because I think, for many questions, the right answer is actually, "Maybe it's this. Maybe it's that." If you refuse to say that, you have convictions and you have commitment, you're committed to saying an answer that's not true, and I think that's bad.
I subtitled this lecture: Mathematics and the Liberal Arts. I want to pivot in that direction, not just because I'm here at a liberal arts college, but because I truly believe that mathematics is one of the liberal arts. I think that's how it functions. I think we're in an era where, in some sense, the idea of the liberal arts is under attack, sadly, partly sometimes by some of our colleagues, of course more the engineers than us, but sometimes ... I just want to pivot now, because, at least for me, how did I, as a college student, learn to start thinking about these things in a way that made sense to me, and it was maybe from a slightly unexpected source.
Okay, this is the hardest of the three. Who's this? You can kind of tell it's a poet from his eyes, right? Look at that piercing, penetrating gaze. This is John Ashbery, one of the greatest of 20th century American poets, and actually still out there making poems for the 21st century, but, like most, rising to prominence in the '60s and '70s. I'm just going to read you a little bit from one of his poems, that I love, that's kind of about this. This is probably the most famous poem he wrote. It's a poem called "Soonest Mended." I don't have the year. I think it's 1963 that he writes this.
He says, "And you see? Both of us were right, though nothing has somehow come to nothing. The avatars of our conforming to the rules and living around the home have made, well, in a sense, good citizens of us, brushing the teeth and all that, and learning to accept the charity of the hard moments as they are doled out, for this is action, this not being sure, this careless preparing, sowing the seeds crooked in the furrow, making ready to forget, and always coming back to the mooring of starting out that day so long ago."
"For this is action, this not being sure." This is something I sort of say to myself, like a mantra. It's exactly the right repast to what Theodore Roosevelt has to say. Theodore Roosevelt would have vehemently denied that not being sure was a kind of action, but I think he was wrong, and I think Abraham Wald and Nate Silver show the exact way in which it's wrong.
The mathematician's not being sure is not the not being sure of just shrugging, right? It's not the not being sure of just saying like, "I don't know." Okay, I agree that's not action, but a kind of principled not being sure, a kind of attempt to tame uncertainty, the way, actually, most of the history of mathematics went by without us being able to do. Probability is a relatively recent part of mathematical history, but now it's absolutely central.
I want to say one more thing about Nate Silver, actually. I'm going to raise the stakes even a little more. Here's another diagram from Nate Silver's page. This one's a little harder to parse than the other one, so what is this? This is showing for each state ... I think this is from around the same time as the other chart I showed. For each state, what is Nate Silver's estimated probability of Obama winning that state. For instance, Pennsylvania, where we are now, he thought that Obama had a 96% chance of winning, a near certainty. A state like New Hampshire, much closer, he thought Obama was ahead, but there was a 75% chance Obama would win, and Mitt Romney still had a 25% chance. On the other hand, a state like North Carolina, he thinks that Romney is very likely to win, 81% chance, but there's a significant, small, minority probability that Obama will win. That's his estimate at this time.
One way of thinking about this is you could say, "Okay, Nate Silver, what's the chance that you're going to get New Hampshire wrong?" "Well," he would say, "Okay, I give Obama a three-quarters chance of winning, so I guess I'm calling it for Obama, but there's a one-quarter chance that Romney actually wins, and I'm wrong there. Okay."
Another way to say that ... Talking about probability always leads you to make weird counterfactuals, so here's a weird counterfactual. What if the election happened 10,000 times. Okay, that doesn't really make sense. To go further into it, we would have to talk about the philosophy of probability and what probability actually is, which is amazing, but also impossibly confusing, and we're not going to talk about it, so just bear with me on this thought experiment and say, well, one way of thinking about this is, on average, every time he won this election, about a quarter of the time, Nate Silver is going to get New Hampshire wrong, so one-quarter of a wrong answer per election.
You can actually do that for all those states. For instance, a state he's more sure about, like North Carolina, he thinks only .19 wrong answer per election. A state that he thinks is very close, like Florida, which remained close right up to the end, I think at this moment, he gave Obama a 59% chance of winning, so he says, "There's a 41% chance I'm going to get this wrong." Then, you could answer the question ... Here's a question nobody asked Nate Silver during the election. If they really wanted to show him up, I think this would've been cool. They could have asked Nate Silver, "How many states are you going to get wrong? Make a prediction of that, a meta-prediction."
Nobody asked Nate Silver that, but, because it's math, we don't need the real Nate Silver. We can just simulate Nate Silver, and we know what he was doing, and say like, if you had asked Nate Silver, "How many states would you estimate you're going to get wrong?" he would have said, "About three." For the technical people in the room, it's because expected value is additive, so I don't have to say anything about Independents. I don't have to say anything about anything. I can just add up the expected values.
Then I think it would have been awesome if somebody had asked him that and then he'd gotten all the states right, and then people had criticized him on the ground of getting more states right than he said he was going to get. That would have been an amazing meta-moment that happened only in my mind. One way to sum up ... It's kind of paradoxical right? Actually, you have to take a moment to be like, that's sort of weird that he's predicting that his predictions are going to be incorrect. I actually think this is an important thing to take some time and think about.
One way to sum it up is a great slogan, which comes from the philosopher, Willard van Orman Quine, where he says, "I always think I'm right, but I don't think I'm always right." Take a moment to think about that. How can you believe that your beliefs are not correct. That seems paradoxical, but it's not, because, of course, if you're honest with yourself, there must be something that you believe that's not correct. Somewhere in there, there's some amount of probabilistic reasoning, something about the uncertainty, which is actually turning into something that looks like a paradox, something that looks like a contradiction.
This talk is called Uncertainty and Contradiction, and I'm now pivoting. I talked about uncertainty. Now I want to up the ante a little bit. There's a stereotype that mathematicians can't handle uncertainty and deal with ones and zeroes, black and white. I hope I've dispelled that.
There's an even more strong stereotype that mathematicians are allergic to contradiction, that we can't handle that. I think that's also wrong. I think the stereotype is that mathematicians presented with contradiction behave something like this. This is, I think, the most outdated of my cultural references in this talk. This is ... Do I have a date? I think this is an episode of the original Star Trek television series, called "I, Mudd," from 1967, so probably around the same time Ashbery's writing this poem. He's probably watching this show. This is an android, whose name I forget, who has been ... Captain Kirk dispatches about like 18 androids in this way, over the course of the series. Whenever a computer gets too uppity, he sort of tells it a contradiction, and then it's head explodes. Someone on the Internet captured this in the moment of head exploding.
It's not just a piece of silly science fiction. It actually reflects something in formal logic, which actually, by philosophers, is called the principle of explosion. That's what it's actually called, or ex falso quodlibet, like from a contradiction, everything follows. A statement like this is, logically speaking, completely correct.
I think I actually have one more proof to do, so I think I won't walk through this. I'll just let this sit. No, maybe I'll actually say it. Okay, so the idea is very simple. It's this. You say, "Okay, so it's true to say that if the Giants didn't win the World Series, I am the Pope," because the Giants did win the World Series. Actually, now I've forgotten if this is one of the years they won. I think it was. Yes, it was. No? Somebody-
Audience: [inaudible 00:29:57]
Jordan Ellenberg: The great thing is it doesn't matter for my deduction, but that implication being correct, then if you throw in the added information that in addition to winning, they also didn't win, then that does indeed imply that I am the Pope, and that is a completely correct deduction in formal logic. Formal logic is, in some sense, very brittle when faced with a contradiction. It completely explodes and falls apart.
People are not like that, even mathematicians. The way I like to think of it was summed up by F. Scott Fitzgerald, who has this famous slogan about this, where he says, "The test of a first rate intelligence is the ability to hold two opposed ideas in the mind at the same time and still retain the ability to function," unlike the android. Fitzgerald did not have access to Star Trek, but he anticipated, by about three decades, what it would be about.
I think actually, in some sense, this is a trick. This trick of holding opposed ideas in the mind at once is something that's very common in mathematical thinking. Sometimes I actually think it's something that mathematicians have to offer the rest of the mathematical arts, by example. The famous example is this. I promised I would tell a story about the Pythagorean theorem. Here's a depiction of the Pythagorean theorem, right? I have a right triangle, with two equal legs, and the hypotenuse, which is unknown. Of course, the great and terrible discovery of the Pythagoreans is that if, let's say, we take X equal to one, then the value of H by Pythagoras's theorem was the square root of two, and what they learned is that that is not a rational number. That doesn't really bother us, but it bothered the Pythagoreans a lot.
The story about it is, of course, that Hippasus, who apocryphally discovered this was apocryphally thrown into the sea and killed, for proving such a nauseating theorem, the point being that somehow for us it's no problem, but for the Pythagoreans, their numbers system really had no accommodation for an irrational quantity, right? For them, a magnitude, the size of a thing, a quantity, just was a ratio of two numbers. For us, it would be like if somebody wrote a geometrical proof that that side of that triangle literally did not have a length. That'd be pretty upsetting, right? That's the situation that they were in.
How do you prove this? Well, I think walking through it step by step is maybe not so productive, but I do like to show it, so we can see that it's not a long argument. Many of you have taken elementary number theory and know it. It looks like this. The only point I want to make about this proof is that it's what's called a proof by contradiction. What that means is we start in what, to non-mathematicians, is a very weird place. We assume that H and X are natural numbers, such that H squared is twice X squared. That's what the Pythagorean theorem demands. From this, we derive a contradiction. That is kind of a weird thing to do. It's not normally what we do when we're not doing math and we think about things.
We don't usually go around assuming that what we expect to be true is, in fact, false, but I kind of think we should, because, in some sense, it's so successful within mathematics. It's a basic tool of thought to put ourselves into this virtual world, where the thing we're trying to prove is wrong, and see what happens. It's like exploring an alternate universe. In fact, this is not what Ashbery was actually talking about, but, elsewhere in the poem, he talks about a kind of "fence sitting, raised to the level of an aesthetic ideal," and this I like very much, because fence sitting is exactly what we're trying to do, trying to poise ourselves between believing a thing is true and believing that thing is false, trying to hold both in our mind at the same time, while retaining the ability to function, as Fitzgerald asked us to do.
In fact, it's sort of a common piece of math folk advice. I know my advisor, Barry Mazur, told me to do this, and he probably heard it from his advisor, and etc., etc., that when you're working hard to try to prove a theorem, what you should actually do is this: You should try to prove it by day, but try to disprove it by night. Again, in a normal line of work, that would be very strange advice. If you're a software engineer, nobody says you should take bugs out of your code in the day and then put them back in by night, but in math, this is a very real strategy that we do.
Why do we do that? There's two good reasons. The first is pretty simple, that it's a hedge against waste, because, after all, you could be wrong about what's true. It could be that you spend all day every day trying to prove a theorem, and that theorem is, in fact, not true. If that's the case, then you are going to fail. You might not know that you're going to fail, but you are, inevitably, going to fail, and you're going to have wasted some titanic amount of your time, so trying to disprove the theorem by night is, in some sense, a hedge against that massive waste.
There's a deeper reason than that, actually. As I said, if something is true, and you try to disprove it, you will fail, right? Failure sounds bad, but failure is not always bad. What happens? Maybe you try to disprove this statement, and you hit a wall. You try to disprove it another way, and you hit another wall. Every night, you try to disprove your theorem and every night, you hit another wall. After a while, in an ideal situation, what happens is those walls start to come together into a structure, and that structure is the structure of the actual proof of the theorem, which really is true, and what you're trying to get. In other words, if you truly understand why you are failing again and again to disprove the thing, this is often the route to successfully proving it.
I think those of us ... I see some nods. Good, that's reassuring. I think those of us who are in the business will recognize this is a process that, whether we formally choose to undertake it or not, is part of the process of generating new mathematics. I guess I actually really do think that this principle is useful, not just for mathematicians trying to prove formal theorems, but, more generally, that it's rather a healthy exercise to put pressure on your beliefs, to believe whatever you believe by day, but at night try to argue against those things. Don't cheat. Really try to put yourself in the position where you believe what you don't believe and see how that feels and see if you can argue yourself out of what you believe by day. If you can, then you've changed. Great. If you can't, then maybe you know something more about why you believe what you believe. I truly think this is a salutary exercise.
"This is action, this not being sure." Part of what we're doing, as I said, is trying to be uncertain or even trying to be contradictory, not in a helpless way, but with a purpose or even ... Whether it's to talk about something that's inherently uncertain, like Nate Silver, or even to somehow use that contradiction as a way to propel yourself into actually understanding something, the way that Hippasus did at the very, very beginning of mathematics. Of course, I'm thinking about Fitzgerald here and his advice. I'm thinking about Samuel Beckett. "I can't go on. I'll go on," one of the most famous expressions of this idea.
Let me just close, because what I really am thinking about here is probably the most mathematical novelist that we have or had, David Foster Wallace, who thought about paradox and contradiction all the time. I think, among contemporary American literary figures, he's the one who's really using this. He's using it outside the mathematical context and in the humanities context and really deriving something from it. I'm just going to read a couple of quotes and then ... because he's such a beautiful writer.
This is when, from his first novel, he refers to the famous paradox of Bertrand Russell. "Lenore was looking at the ink drawing on the back of the Stonecipheco label that lay on the top of the notebooks in the desk drawer. It featured a person, apparently in a smock, in one hand a razor, in the other a can of shaving cream. The person's head was an explosion of squiggles of ink." See, the exploding head is a common feature again and again.
"I think this guy here," Lenore says, "is the barber who shaves all and only those who do not shave themselves." The big, killer question is supposed to be whether the barber shaves himself. I think that's why his head's exploded here. If he does, he doesn't, and if he doesn't, he does. This is a central figure and a central motif in Wallace's first novel, The Broom of the System.
Let me read one more. This is from his later short story, "Good Old Neon." He says, "There was a" ... Where he expands that out. In his early work, I think he's going to be using mathematics as just something that he knows about, and most novelists don't, and he's sort of putting it in for color. Later, I think it integrates more fully into his writing about the human condition, and he writes this.
He writes, "There was a basic, logical paradox that I called the fraudulence paradox, that I had discovered more or less on my own, while taking a mathematical logic course in school. The fraudulence paradox was that the more time and effort you put into trying to appear impressive or attractive to other people, the less impressive or attractive you felt inside. You were a fraud, and the more of a fraud you felt like, the harder you tried to convey an impressive or likable image of yourself, so that other people wouldn't find out what a hollow, fraudulent person you really were."
I guess I started on a downer and I'm ending on a downer. Truly, but I mean, you see here someone struggling to do what I think, in a liberal arts institution, we want to do, which is to truly live the ideal that all of these things we're learning, all the parts of the intellectual heritage that we have, are supposed to work together. They're not supposed to be in separate classes that are divided by buildings and divided by discipline. They are all one thing. I think it's actually not too strong, when I make this claim for David Foster Wallace, that his writing was driven by his struggle with contradictions of a logical time. He was in love with the technical and analytic and starts in the middle like a philosopher, but at the same time, he saw that the rather simple statements, offered by religion and by self-help and by Alcoholics Anonymous, offered better weapons against drugs and despair and killing solipsism than all of the logic that he knew.
He knew it's supposed to be a writer's job to get inside other people's heads, but the chief thing he wrote about was the predicament about being stuck inside your own head. He was obsessive, actually, about recording and neutralizing the influences of his own preoccupations and his own prejudices, but he knew that that determination was one of his preoccupations and one of his prejudices at the same time. Okay, all this stuff is, in some sense, part of Philosophy 101, but one thing we learn in math is that the problems that you encounter in your freshman year of college are actually some of the deepest problems that exist, that we never really fully understand.
David Foster Wallace, I think, wrestled with the paradoxes in much the way that mathematicians do. You find yourself believing two things that seem to be in opposition. This happens to us a lot, and so you go to work, step by step, individual logical steps. You clear the brush. You separate what you actually know from what you believe. You hold those two opposing hypotheses side by side, viewing each one in the adversarial light of the other until the truth, or as close as you can get, comes clear. I'll stop there, with this picture of David Foster Wallace. Thank you very much.