Lynne Steuerle Schofield '99 - Lecture
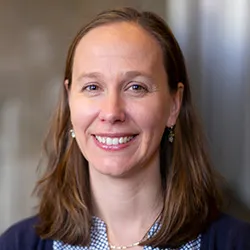
In her talk, "Tests: What are they good for?", Lynne Steuerle Schofield '99 discusses latent constructs, which are variables that are not directly observed, and how statisticians can attempt to measure them.
Associate Provost of Faculty Diversity and Development and Associate Professor of Statistics Lynne Steuerle Schofield '99's interdisciplinary research includes areas of mathematics, primary, secondary and tertiary education, environmentalism, cognition, public health, and public policy. In 2018, received the Waller Education Award from the American Statistical Association in recognition of her outstanding contributions to and innovations in the teaching of elementary statistics.
Audio Transcript
Lynne: Hi, friends. I think we'll get started. Those of you that don't know me, my name's Lynne Steuerle Schofield. I'm class of '99. It's my 20 year reunion, and I now teach here at the college in the department of math and statistics. I also serve as the associate provost. So, can't quite get away from Swarthmore, but there you have it.
So, I want to start by just saying that there's such a small group of us that if, as much as possible, let's try and make this a discussion. So, as I'm saying thing, if things don't make sense, please feel free to interrupt me, raise your hand, ask questions, do whatever you need to do if something I say doesn't make sense. I left about 10 minutes here at the end for questions if you have any, but feel free to just interrupt me. I'd rather us have a discussion as we go along.
So, since we're at a reunion, I thought it might be a good idea to start by kind of going back in time. I wanted to go back farther than the 20 years since when I graduated, closer to class of '89, '94. So, when that group of people were graduating from college, I was in middle school and high school. One of the things that I did in middle school and high school was that I spent my allowance on 17 Magazine.
Now, part of the reason for this of course was that I wanted too, to understand how to have a year of great hair. I thought it would be really great to know what the 45 coolest things to do this summer might be because as you can imagine, someone who's going to grow up to become a statistics professor is not what most middle schoolers think of as cool. So, I thought this might be a good way to learn how to be cool. But I found that when I would get my magazine, I would not turn first to the articles about how to have great hair or cool things to do, but instead I always found myself turning directly to the quizzes.
For those of you that have never seen any of these quizzes, here's a couple of examples. They are really pretty amazing, right? So, you can learn things like, "Do you know when a guy is into you," that helps you determine whether or not you're a blind to love babe, whether you're a man-reading mama, or whether you're confident but clueless. You could also learn if you were a risk taker, if you were a daredevil, if you sometimes take risks, or if you play it too safe. These were sort of the important things that 15 year old Lynne really wanted to know.
So, the nice thing for today's middle schoolers and high schoolers, and I have a middle schooler myself, is that you don't need to spend your hard earned allowance on 17 Magazine anymore. You can go to Buzzfeed and you can learn things like what Harry Potter character are you. And here, if you learn what Harry Potter character you are ... Oh, why isn't this showing up? Right? You can answer important questions like what your favorite movie is or what object you most desire, or even your favorite food. You'll learn incredibly important things by discovering what Harry Potter character you are, just by answering these questions.
What I found though, is that once about a few of these quizzes and tests went by a few months of getting them, as much as I still really loved taking all of the tests, I found myself starting to ask questions, like who wrote these tests, how did they construct them, how did they score them, and how did how they score them possibly affect the kinds of answers that I would have? So, I would find myself trying to break down the tests and break down the quizzes, and see I'd answer it this way, would I have gotten a different result? Or, if I had done it in this manner, what would have been different along the way?
Now, you might think, okay, that's great, 17 quizzes, who really kind of cares about that kind of thing? But it turns out that the same kinds of questions about who sort of who constructs tests, how they're scored, turns out to play a really pivotal role when you think about the kinds of educational testing that we do, right? So, who constructs the SATs? How do they score the SATs? Who constructs things like the GREs?
Here in Pennsylvania, all high school students now have to take what are called The Keystone Exams, and if they don't pass those exams, it will imply that possibly they won't graduate from high school. K through 12 kids, third through eighth graders and eleventh graders all have to take the Pennsylvania State Scholastic Assessment through the PSSAs, and in order to take those .... how the students do on that test can determine things like teacher pay, it can determine the kind of funding that schools get, it can determine whether in the city of Philadelphia, whether the state decides to take over the school district as a way to handle things.
So, since these tests play such a pivotal role in the kinds of decisions that are being made, the same kind of questions I think, for me, come up. Who designs these tests, how are they constructed, how are they scored, and how would a different scoring method or different way of thinking about it possibly affect the kinds of scores that you get? And it doesn't just happen in educational cases, right? If any of you have ever at any point gone to see your PCP or psychologist and you've said, "I'm concerned that I might be depressed," you might take something like Goldberg's Depression Survey, where again there's a set of questions that you have to answer. And how this test is scored, how it's constructed, can determine the kind of diagnosis you get, which can determine the kinds of resources that you get, whether that be psychological help, whether that be medication that is funded by your insurance, all of these various other things.
So, imagine my surprise when I started working at Swarthmore, and suddenly I'm the one writing and constructing the tests, and I'm the one deciding how it is that I'm going to score them, right? And this means, of course, that if I score them in certain ways, my students might get an A or an A minus or a B plus. And this is only measuring a very specific aspect of all of the things that I see, what I'm doing with the students today.
So, quizzes and tests and all of these different surveys, they measure what we statisticians call latent constructs. So, what do I mean by that? So, latent construct is essentially a hypothetical undirectly measurable that's out there. So in comparison, something like height, right? I can pull out a ruler and I can measure directly all of your heights, and there's a scale of that height which absolutely makes sense to me. If something is six feet tall versus something is three feet tall, we can say the six foot tall thing is twice the three foot thing. We can put two of those three foot things together and we can get something that's six feet tall. If we say measure your temperature, there's a natural scale there. In Celsius, we say that zero degrees Celsius means the freezing point of water. 100 degrees Celsius means the boiling point of water, right? These are things that have physical and natural meanings.
However, something like a test or a quiz, or a latent construct I should say, really doesn't have a natural meaning. What does it mean for you to get 105 on an IQ test versus 100? That doesn't necessarily have any meaning unless we attach some kind of meaning, attach some kind of scale to it. And because we can't observe it directly, it's incredibly difficult to measure it accurately.
So, there are two ways that we score tests in sort of the modern era. The first is what's called classical test theory, or CTT. This is the way that all of the tests that any of you who ever took something in classroom scored. Essentially, we have J items, that's what the X's are. So, X1, X2, all the way up to XJ, those are each of the items. These are the that are answered last on the test, and each of those X's is either a one if you get it correct, or a zero if you get it wrong. Or if you want to make it a little more complicated, it's a one or it's a one half if you get half credit, and so on and so forth. And that theta with the little hat on top, that we call theta hat, is an estimated test score.
So, what do we do? We take all of the score that you get on each of the items, we add them all up, and there is the test score. That's what I write down in my grade book whenever I give out a test for an exam at the beginning or the end of every semester. We think of this of a form of what we call dimension reduction, right? Because essentially what we really have for each individual I, for each person I, we have J pieces of information. But we're going to sum them all up together and we're going to make one thing there on its own, because each of those J pieces of information is just a tiny little piece of whatever it is we're trying to measure. So as a statistician, I'm trying to measure your statistical ability. Each of those is one little piece of your statistical ability and how well you can do that.
So, the key idea, the sort of main amazing thing that happens when classical test theory came around, was that they were the first individuals, those who developed CTT, were the first individuals to recognize that these test scores were really just estimates of this broader construct that we were trying to measure on some level, right? So if again, if I'm measuring statistical ability, the theta is the actual true score. It's whatever your actual statistical ability is, and theta hat is just my estimate of it. And so, that little epsilon up there at the top is the error between these two.
So, this was really kind of the first group of people to say these things are latent constructs. The test itself isn't actually the exact measure of your latent construct, it's just an estimate of what it was. So, that was kind of mind blowing around the 1940s, 1950s. There's three problems with this method though, of our way of scoring, of our way of using it.
The first is that it's what's we call unidentifiable. So by that, what I mean is the only thing that we observe is your theta hat, and what we want to know is your theta, right? So, let's say that I have a theta hat of 75. What I don't know is whether your theta is actually 100 and the error is negative 25, or whether your theta is actually 50 and the error is plus 25, right? And it could be any infinite number of things that theta and the error could be together because we only observe the one thing, but we've got two things that those two things are summing up to. And so, unless we have some what we call untestable assumptions, we can't really determine anything either about theta itself or about the error that's associated with it, and that can be problematic.
Second with classical test theory is that it turns out it's pretty impossible to compare two examinees who take two different tests, right? So, when I give my, say Stat 11, which is a class we teach a lot of here at Swarthmore. It's our stat methods class. It's probably a class some of you took at some point. When I give that at say the fall semester, and then I reteach that class and give a different final in the spring semester, sometimes the fall semester I write a harder final than I do in the spring semester. So, if I take a score of 75 on the fall test and a score of 75 on the spring test, that doesn't necessarily mean the same thing because they're two different tests, and in some sense there's no way for me to separate the test score from the examinee itself. I can't separate what parts of the test made it hard and what parts of the score that I've got are in fact about the examinee themselves.
The other thing that turns out to be really important is that the match between the test and the examinee becomes incredibly important. So, let me give you an example of what I mean. I'm guessing that if I handed out to all of you a first grade math test, you'd all do really, really well on that test. And I'd have no way of really distinguishing among all of you which ones of you were like me, were a math major here at Swarthmore, and which ones of you avoided math at absolutely all costs while you were here and you haven't studied it since you were high school seniors. I'd have no way of being able to separate that out based on a first grade math test.
Similarly, if I gave all of you, say, the final to, I don't know, our real analysis seminar, right? Which is one of the hardest classes here at Swarthmore in the math department. Again, that's not going to separate out those of you that used math in some other form versus those of you that do real theoretical math. So, the match between what it is we're trying to measure and actual sort of ability level of individuals turns out to be super important along the way.
So, around ... I'm going to say about 1960 or so, a group of individuals developed what's called item response. It's a second method to scoring tests. Now, item response theory is, as you would imagine by the name, item oriented. So, the key idea of what happened here is that the individuals who developed IRT essentially said what we'd like to do is actually look at the fact that each of these items are giving us a little piece of information. And so, rather than take the test score, let's look at seeing if we can actually figure out certain things about each item, based on item information itself.
So, a typical model mathematically graphs out to being something like this. So, along here on the X axis we have the ability, okay? So, I've made ability run from negative four to four, so that zero you would be of average ability, whatever that is. So, if you're in the negative, you're sort of below ability on whatever I'm measuring on this test, and if you're positive, you're above ability. On the Y axis, what we're going to actually try to measure is the probability that you get a particular item correct based on your ability, okay?
So, that S curve is a pretty typical curve for what these look like, and the reason for that is if I have a test question that's for someone of about average ability, if you're really, really, really low ability versus only really low ability, probably that question isn't going to distinguish very much between the two of you. So, your probability isn't going to go up particularly high if you're down here on the scale. And similarly, if you're really, really high ability and you have a question that's say, kind of about an average question, that question isn't going to do a good job of distinguishing among. So, where it's really going to distinguish, where you're really going to see huge differences in the probability of getting the question is going to be right around where the ability and the test question itself match.
So, this IRT model is a function of four things. So, it's a function of the individual's latent trait of their ability, of their depression, of whatever it is that we're trying to measure, but then it's also a function of three characteristics, if you will, of the item itself. So, those three characteristics include what we think of as the guessability of the item. So, how easily can you guess the right answer, right? If what we're trying to model is the probability that you get a right answer, it's a multiple choice question and you have no idea what it is but there's four possibilities, you still have a 25% chance of just guessing randomly to get it right. So, we want to model that in some way.
It's also a function of the item's difficulty, right? So, how hard is the question versus how easy is the question? Questions that are really hard, they're going to have lower probabilities of getting it right for sort of the average person along the way.
And finally, it's a function of what we call the item's discrimination. And I don't mean discrimination as discrimination in racial discrimination or gender discrimination, I mean it's ability to discriminate between low ability an high ability. The key part of item response theory is now that we've got these many measures and now that we know these various characteristics of the items, is that we can now actually separate the things that make the probability high for an individual to get an answer correct that are based on the individual themselves and the things that are based on the item itself, on the test itself, along the way. And because we now have several different measured of an individual's ability, of an individual's latent trait, we can also measure the error that's associated with that test along the way.
So, for those of you that are mathematically oriented, here's what sort of the mathematical model actually looks like of the typical IRT model. And again, just to sort of be really clear, we're measuring the probability that person I gets question J correct, which is what that X=1 is. That's going to be based on the guessing parameter, which is the CJ, the difficulty parameter, which is the BJ, that discrimination parameter, which is AJ, and theta, which is that cognitive ability or that latent trait of the individual.
So, just to give you some sense of how these item parameters kind of change the way these curves look of these models, these are three different items that we might have, right? So, the blue item, that has a higher guessing parameter. Ans so, what that means is that that's going to change, for a person with low ability, that's going to change what we call the Y intercept, right? Just automatically, that person has a 20% chance. Whereas question red and question black, this is probably not a guessable question. Maybe this is an essay or a short answer kind of question that we would have, right? So, that's going to change what we call the Y intercept, and make it go up and down depending on what that value is.
The difficulty parameter is going to tell us something about where the location of it is, okay? So, the blue is the easiest question because there I only have to have an ability of about negative one in order to have a 50/50 chance of getting the question correct. Whereas the black one, I have to have an ability now of zero, right? Which is sort of my average ability in order to have a 50/50 chance of getting that correct. And the red one, I've got to have an ability of one, right? So, one whole standard deviation above in order to get that one correct. So, that affects the location of where we see things along the map.
And finally, that discrimination parameter affects the slope, right? So, the higher the discrimination parameter, the steeper the slope. Because remember, we were saying that's the one that tells us about how well it can distinguish low ability and high ability individuals. And so, the blue parameter is the one that has the steepest slope. It's the one that's best able to distinguish people of low ability, which in this case is about a negative one ability, versus people whose ability is above negative one. Whereas the red one is not really as good. You can see that I have about a 40% chance of getting it correct when I average, but that only goes up to about a 60% chance going all the way up to a score of two, right? About two standard deviations above. So, it doesn't do quite as good of a job. Okay.
So, there's two kinds of questions of interest that these tests might be able to help us answer, right? So, besides the fact, as I sort of mentioned in the very beginning, might want to be able to do things about figuring out how we're going to use these tests in some ways. And so, the two kinds of questions, if you will, the two kinds of things that we tend to be trying to answer are questions of inference interest, which I'll talk about first, and then questions of where I might be trying to use the tests to predict something.
So by inference, what I mean is we're trying to, in this case, actually understand the causal mechanisms between these various latent traits. Whether they're cognitive ability, whether they're what economists call non-cognitive traits ... things like personality traits. This might include grit, it might include conscientiousness, motivation, these kinds of things. We're trying to understand the causal mechanisms of how when we develop these at a young age, what that means for later life outcomes along the way. The goal in these cases is to sort of be able to inform public policy and educational practice. This is where a lot of my personal work falls.
Okay. So, here's an example research question that comes from a paper that I worked on with two co-authors, Brian and Taylor. We wanted to know whether blacks attend college at lower rates than comparably skilled whites, and that's kind of the key part. That's why I italicized that, right? So, we can look at demographics to say do blacks and whites attend college at the same rates, and they don't. On average in the United States, whites attend college at higher rates than blacks do. But what we'd like to be able to do is break down and understand a little bit more about why, right? Because this is the kind of thing that's going to inform our public policy and going to inform our practice.
So, we want to know is this gap in who attends college, is that due to differences in math ability prior to entering college? If that were true, right, then what that would suggest is that our public policies and practices, either at the college level or at the K12 level, should attack issues of the fact that there might be blacks on average who have lower math scores. We might want to work to figure that out, to raise those scores, to make them comparable with one another.
Yes?
Lynne: Right. So, what I would do is I would start by looking at whether or not they had the same probability of attending college without comparing people of the same math skills. Then if I compared people who had the same math skills and I found no gap anymore, that might suggest that in fact the gap was due to those math skills. So, it's almost like comparing what's going on without looking at maths comparably skilled individuals, and then looking and saying if I do look at only comparably skilled individuals, does the gap go away? Does that help? Come back to me if that didn't help at all.
Yes?
Lynne: Nope, it's not, but these are just sort of two examples of what I was sort of suggesting that we would want to do. But we were particularly interested in the comparably skilled, right? So, we're really interested in this top part. So I'm saying is this what's going on, or could it be some other thing like financial constraints, like some kind of college practices, other kinds of things that go on along the way, right? So no, these are not the only two things. There are tons more that we might want to test. We were mainly testing the top one in our paper that we were doing.
But I think the main point is to recognize that sort of the decomposition of the gaps that exist. We want to be able to decompose those into which parts of them are based on the fact that individuals have different ... are not comparably skilled, versus which parts of them still exist after we allow for the fact and control for the fact that the skills gap might exist as well. We did not in this, but you could also look at that. Yes, absolutely. For this paper, what we had were scores on a math test, and so that's why we used the math test scores. That's the data that we had. Right.
So, different answers to these questions of course are going to lead to different educational practices. Swarthmore College might do things differently, right? If we knew an answer to this question, based on whether or not one of these two things were the differences, or any of the other kinds of things that could be happening. We could also have different interventions, we could have different policies.
All right. The second example, this is an ongoing project that I'm doing. So, I'm working with some medical practitioners, a demographer, and well as a biostatistician and and economist, and we're looking at scores on what's called The Montreal Cognitive Assessment, the MoCA, which is the test that doctors give to individuals they are concerned might have early onset Alzheimer's or early onset dementia. And so, the data that we have is scores for a group of individuals on the MoCA, and then we have information about them five years later and a retest of them on the MoCA. Then we have again another test five years later of this.
And so, what we're trying to look at is whether or not knowing something not just about the MoCA 10 years, say, prior to someone being diagnosed or not being diagnosed, combined with some other measures of the test, which include things like the response times. So, not just whether you got the answer right, but how long it took an individual to answer a question on the test. Whether we can combine those together to actually get earlier estimates, earlier predictions of whether an individual is going to develop early onset Alzheimer's or early onset dementia in such a way that this can be useful to medical practitioners, right? Because if that's true, we might be able to develop earlier interventions that might necessarily lead to sort of better outcomes, whether those outcomes are a longer life, or at least maybe not a longer life, but at least a more appreciative life for while we.
But again, what we need in some sense in some measure of cognitive ability, and we need to be able to do that and put that into a model where we're predicting something else, whether or not that individual is going to get that, and understand how that assessment infers something about what's going to happen later on.
Okay. So, most people when they use these kinds of questions, right? When they have a test and they have something they're trying to predict, and they're trying to understand the way in which the test score can infer certain things about what's happening later on, is they just take the test score as a proxy for that latent construct on its own. Turns out, this is hugely problematic. Why?
Well, most of the way that we do these kinds of things is something called regression analysis. So in a regression analysis, I'm going to take a whole bunch of individuals. So, every dot on my graph here is an individual within the data set that I have, and I have some measure X. This might be their test score, right? So, this is a test where you can get it up to 100, and I guess the lowest score someone got was about a 62, right? So, this might be the test score that I have, and on the Y axis might be what it is that I'm trying to predict. So, let's say I'm trying to predict, I don't know, weekly wages or something like that, right? So that would say that this person got a 68 or so on my test, and their weekly wages are about 190, right? In comparison to a person up there who got a score of about 95, and their weekly wages are closer to 325.
Okay. So, what regression analysis does is it tries to build a mathematical model where what we assume most often is a linear relationship between X, or our test score, and Y, our wages. What we're going to do is try to draw what we call the best fit line through that set of dots to be able to say something. And what the dot does, or what the line does I should say, is it gives us some sense of what the slope is. What the slope is, is it tells us if I increase someone's score by 10 points, right? If I increase their score by 10 points, then that tells me something in the vertical direction of how much their weekly wages go up, right? So, if I can get everybody's scores higher, then maybe I can actually get all their wages higher because it gives them some additional set of skills along the way, right? So, these are the kinds of things that we might look at.
So, most common, it's common to assume linearity, although we don't have to assume linearity, right? We can make other assumptions about the functional form, but we're going to stay with linearity for here. But the key assumption in here is that X, whatever is on this line down here, has no error associated with it at all. That's the key part. The only place where there might be some error is in Y, in comparison to what we would predict, which is where you would be on the line versus what we actually observe. So, we assume no error at all in X. This is problematic because, as we've talked about, test scores are what we call noisy, right? They have error associated with them.
So, what are the kinds of errors that might happen? Well, all of us ... I remember when I was a junior in high school, I took the SATs and I had bronchitis. Let me tell you, I did not do very well. And I'm pretty sure that because I was coughing a whole lot, no one else in the test center did very well either because it was really annoying to listen to me cough all the time. It's probably pretty distracting, right? It can also be too cold or too hot in a test, right? So that you're not really sort of always able to do the very best that you can. It may be that you studied the wrong set of things, right? And so, in fact you 90% of whatever it is that somebody is trying to test you on, but they only test you on the 10% that you didn't know. Or vice versa, you only know 10% of what happened, and that's the 10% you got lucky that the test asked you, whereas you actually don't know any of the other 90% because you just happened to study that particular area along the way.
And so, all of these things are going to lead to the kind of errors that we talked about before. Those epsilons we said exist. So, when we have noise in the X, here's what happens with the regression. So, for every one of my black dots, I added some error of some kind, and I added random error. So it could either say for this person, their test score was about 79, right? Or, I'm sorry, their true score is about 79, but we got on their test score, what we observed, was about 82. Versus that person up there, their true score is about a 70, but what we observed here was about a 51, right? So, we see all of this kind of error.
So, the only place where we're seeing error is in the horizontal direction, right? So, what you see is that sort of spreading out of my data. That's spreading things out in this horizontal direction. So now when I'm trying to draw the best line, right? What's going to happen, instead of the line being like this, in order to get all of the data within here, it's going to shift the line down. And so, suddenly the effect, of say the test score on wages, is going to look smaller than it actually is. And you can have error going in the other direction as well. You can actually have error that attracts things in certain ways that will make it look too high. But this just gives you some kind of idea. So, when we have this test score error and we try and throw it in without thinking about that error at all, it poses a problem. It poses issues with our ability to actually understand the relationship that exists between X and Y.
Here's what makes it even more problematic. If we have other predictors in the model, right? So if there are other things that we think might affect, say, your wages, or might affect your ability to go to college, like race or gender, if those other things are also correlated with your test score and you don't account for the error, then the effect of those other things on whatever it is you're trying to predict will also have buy in. And so, our ability to actually measure racial differences when we put something like a test score into our model, as long as we don't account for that error, it's going to be problematic not only for understanding the relationship between the test score, and say wages or likelihood of going to college, it's also going to pose a problem for our ability to estimate the racial gaps, the gender gaps, or any other thing that we think might affect whatever it is that we're trying to predict. Yes.
Yeah. So, you would also have to somehow account for the fact that you probably ... yes, exactly. You have selection into certain classes based on various characteristics, right? Some of those are going to be geographic characteristics, right? And since people in the US tend to live within race and tend to live within socioeconomic status, you're going to see these kinds of selection things are going to play a role as well. This model isn't going to account for that. There are some ways to account for that in some sense, which some of those value added models attempt to do, but every time you're sort of playing catch up on trying to control for all of the things that these selections.
So, what my colleagues Brian Taylor, and Dan Black and I realized is we have to model the error, and we suggest a way to do it. Now, I'm not going to actually go through all of the mathematics of how we suggest doing this, but the basic idea is that what we say is you have to simultaneously estimate the error within the test score at the same time that you're estimating this relationship along the way. So, it requires a lot of computer power and it also requires that we actually know the answer to every item on your test, not just your overall test score at the very end. So, it's a data hungry kind of model in that sense.
So, let me just give you some sort of example of how much of an effect not modeling versus modeling the test score can have. So, this is from that same paper that I mentioned here, that Schofield, Taylor, and Black, 2015 error. Here we were estimating the odds ratios between whites and blacks of going to college, on racial of going to college. So, when we looked at comparably skilled individuals going to college and we did not measure the measurement error, the odds ratio is said that whites have 1.27 to 1 higher odds of going to college than did blacks for men. And for women, it was about a 1:1 ratio. The 1.27 to 1 was what we call statistically significant, meaning we sort of ... even within our sample, we would expect to see the same thing with this population, that there would be this difference along the way.
When we modeled the measurement error in here, what we found is that in fact, the odds ratio greatly decreased. And so, instead of it being 1.27 to 1, it went to 0.98 to 1. Now, that's not statistically significant, but what that's essentially saying is that in the first case, we thought whites were far more likely to go to college than blacks, compared to skilled blacks. When we modeled the measurement error, we're actually suggesting that whites might be slightly less likely to go to college than comparably skilled blacks. And for women, we actually found a statistically significant difference for black women who are comparably skilled to their white counterparts, are in fact more likely to go to college.
So, just modeling the measurement error gives us fundamentally different results in the kinds of ways that we would think about what are the things that we need to do. And because we know ... and this sort of goes back to your question, because we know in fact in the population, black women do not go to college at the same rates as white women do. But once we account for comparably skilled individuals and see that black women are more likely to go to college as their comparably skilled whites, this would suggest that at least in this case, a huge part of that gap is because of in math fields.
Okay. So, I'm not the only one who does this kind of work, right? There's lots of other individuals that are interested in it, and it's all the way back. So, Neil and Johnson are two economists that in 1996 looked at black wage gaps, and they found that when they used the Armed Forces Qualifying Test ... which is the test that individuals have to take in order to actually get into the armed forces within the US. So, this is a pretty good test. That when they used that, they demonstrated the importance of racial differences in these scores in shaping the black-white earnings gap, essentially seeing that a lot of the black-white earnings gap could be relate to differences in black-white skills.
I've also looked at the the role of prior math skills and personality traits. So, things like an individual's extroversion versus introversion, their openness to new experiences, what are called The Big Five in psychology, at looking at STEM retention gaps. So, STEM is science, technology, engineering and math. So, whether or not individuals who came into college saying they were interested in studying sort of the sciences, those retention gaps that exist between under-represented minorities and whites, and blacks and whites, and find that math skills play a huge role in the differences between individuals of different races in staying within STEM. And the personality traits play huge roles in staying in ... for men and women. I learned that women who are less agreeable to more likely to stay in STEM. So, apparently I'm not a very agreeable individual.
Okay. So, those are the kinds of inference questions that we want to ask, right? Where it turns out to be really important, this measurement error that you have. But the other kinds of questions of interest that people tend to use these kinds of ideas, these test scores things for, are what are known as predictions, right? So, if I know something about an individual score at an early age, or an individual score on one thing, does that allow for me to predict something about them? Some current or some future characteristic about them.
So in the academic literature, we see things like, who use mother and teacher behavior ratings of aggression and other things to determine whether or not they can predict who is likely to actually commit a crime as an adult, right? So they're sort of trying to do those sorts of things, again, with the hope I think of informing policy and practice to say if there's certain individuals that we think are more likely to commit adult criminal activity at a later age, is there something we can do you at a young age to pull them off that track?
But the other place at this turns out to be kind of big is in Netflix. Whether not ... right? So, last night I got home kind of late and was tired, and what I did was I hopped on my couch and I turned on Netflix, and I said, "What does Netflix suggest that I would like to watch?" Right? What does it predict that I'm going to like based on other things that I have? Oh, this movie looks good. And guess what? I enjoyed it. I had a good time last night. I laughed quite a bit.
And it turns out that Cambridge Analytica used kinds of these kinds of models, albeit not ethically, in the 2016 presidential election. So, let me give you a little bit of an idea of the Netflix model itself, just so you have some understanding. They use slightly different models then these IRT models I talked about. So in 2006, you may all know that Netflix offered a $1 million prize to whoever could make their prediction model better. And they said better buys sort of their accuracy of their recommendations by 10%. Okay? So, I'm giving you a very simplified sort of level of the model that they use because of course it's proprietary and I don't actually know all the details, but this is kind of the basic idea.
So, they use what's called singular value decomposition, sometimes referred to as factor analysis, and it's sometimes referred to as principle components analysis as well. This is, in some sense, another form of dimension reduction, right? So just like those classical test theory models, we're kind of trying to take lots of individual measures of small things and put them down into one particular component. That's the same thing that's happening here. All right. So, I don't actually have Netflix data, but I have something that's kind of similar, right? So, this is data where each individual ... so, each row here that I have is an individual person along the way, and each column is a particular measure of a small measure of that person's body size.
So, ELB.DI is your elbow diameter. So that's literally kind of measuring from here to here along the way. WAI.GE is my waist girth, right? So, that's going to be sort of if I took and found the circumference are on my waist along here, right? So, I've got elbow diameter, wrist diameter, knee, diameter ankle diameter, waist, naval, hip girth, thigh girth, bicep girth, right? So, how strong here is. Your forearm girth, your knee girth, and your ankle girth. There's actually lots of other ones along here as well.
So, I've got all of these measures for every individual, right? So, each of these as a measure for each individual along the way. So in some sense, just like that classical test theory where I had sort of a score on every one test that was all a measure of one particular thing, or statistical ability or depression, whatever it is, these are in some sense all different measures of your body dimensions along the way, of a particular person. So, in singular value decomposition, what we're essentially looking for are correlation among these variables. Which of these values move in the same directions as all the others?
Let me give me you an example of what I mean. So the waist, navel and hip girth, right? So, that's going to be your waist here, where you're going to measure right wherever your bellybutton is, and then your hips right here, right? So, these are going to be the girths right here, which you would imagine are going to move in probably pretty similar directions, right? So, if I'm wider here and I'm wider here and I'm wider here, sort of on average, people in their waist are probably going to also be wider in their hips, on average, along the way. But what we also were looking for is not just sort of across different variables how these things are correlated, but how these movements might differ for different individuals. Are there ways that we can cluster individuals in some way based on how they move?
And so, if we look at the four that I've highlighted here, what you'll notice is that the waists measurements in each one of those ... these are waist measurements in centimeters, are all about 20, 25 centimeters below with the naval and the hip measurements are. If you look at the ones not highlighted, those are all pretty close, right? Those are all pretty even across the way as we look at that. So, what we'd like to do is determine if there are latent variables in this data as well, right? Because each of these are sort of small individual aspects of all of those. So, what the model essentially does is it spits out weights of what we're going to multiply each of the variables that we have, so that we can sum up these various, what we call, principal components or factors, or these latent constructs.
So, I've just given you two here, right? So, principal component one, those are all the weights down this column that I'm going to multiply each of the variables that I have, or the measurement that I have for each individual measurement, and I'm in a sum up those weighted values. In principal component one, I'm going to take each of those ... these are all about negative two. I'm multiply negative two, about negative two by everyone of those, and then I'm gonna sum up what I get when I multiple negative two by each one of those measurements along the way.
In comparison, I'm going to do something slightly different for principal component two because I have a different set of weights here, right? So here, I'm essentially going to add positively, the elbow, the wrist, the ankle, and the biceps and the forearm. I'm going to add all of those sort of like a positive measure within in the second principal component. And the measures that are negative, like the naval, the hip, the thigh, and some of these other ones, right? Those I'm going to sort of have those measure as a negative value along the way. So, does everybody kind of getting what it is we're doing? We're sort of taking these summed values and we're putting different weights on them, so that we're coming up with two different variables, if you will, right? So, principal component one, which is in some sense this kind of negative two times everything. So, it's sort of like an average of all the variables that I have versus principal component two, which is actually separating out variables based on different. Okay.
So, if I plot the values I get for each individual, right? So here and right here, this is the value that I got for this individual on principal component one in comparison to their value that they get for principal component two. Okay. So, I plotted all of this, and what you can think about is that in some sense principal component one, in some sense measures the latent variable, just overall body size, right? Because it's giving each one of the measurements that you have, in some sense, the same weight. So, it's modeling overall body size, but because we were always multiplying by negative values, any negative, any person who has high negative values in principal component one, is going to be a bigger overall person than anyone whose value is more positive on principal component one, is going to be a smaller overall person.
If you remember here, principal component two, the positive ones that we had, right, were about the elbow and the wrist and the forearm. So, they were kind of about your limbs, if you will, right? The values that were along your limbs versus the negative values were really these ones that were your hips, your thighs, and everything else. So, the way that we can think about principal component two, in some sense, is about whether you're more sort of pear-shaped, right? Do you have more weight ... do you carry it here, or do you carry it sort of along your limbs along the way? And so, when we model these two things against one another and then we look at something like whether you're a man or woman, you can see that these principle components actually now cluster individuals into these two different sexes.
And these kind of makes sense, right? Men, on average, tend to be more larger than women on average, although of course there's this huge group in the middle here that we kind of ... right? Tall women or large women, and smaller men. But the other thing that turns out to be true, right? Is that the women tend to have, right? Biologically, we're going to give birth. So, guess what? Our hips and our waists and our thighs tend to be a little bit larger, right? We tend to be sort of more pear-shaped so that we have enough room to push the babies out, and men tend to have things a little bit more proportioned throughout the whole body. And so, this actually allows us to separate individuals into these different clusters based on different things.
So this is body size, but Netflix essentially does the exact same thing. Instead of the date of being body size, right, with they have is again, all these individuals. Then they have things like ratings that people give to different movies. Now, there's going to be a lot of spaces where they have no rating whatsoever at all of your particular individual, but once we start looking at these, what you'll see is that Netflix is going to have things start to cluster. So, it's going to cluster all action movies here together on one part, and maybe a whole bunch of romantic comedies over here.
And so, now it's going to look at what you picked to watch, what you ranked relatively highly along way, and it's going to say, huh, if you picked a bunch of these that you ranked pretty highly, we're going to suggest other ones that fall into that cluster that you never chose before. And so, really, at this very simple level, they can both predict what each person is going to rank other movies, right? That they might like or not like, and it's going to be able to move these movies into various factors or principle components.
So what did Cambridge Analytica do? Well, the Trump campaign used them. They had them consult for them, and they had data that they got from 50 million Facebook users. They targeted digital political advertising, and they used this quiz app called This is Your Digital Life. So essentially, they had a bunch people who took some personality tests, and because that personality test said anybody your friends with we can grab all of their data too, they illegally and unethically grabbed data from 50 million Facebook people. But, they have this very similar model to the Netflix model because what they now have is like data from everything on Facebook.
So again, that same big huge matrix that I showed you before got a bunch of individuals, and now what you have along the top are different things that people might've liked on Facebook versus not. And so, by a same or very similar kind of model, they can cluster these things into what are some other things that we can predict that an individual might like, and that allows them to target the kinds of political ads that they might send. And Cambridge Analytica is not the only one that's doing this, right? Anywhere there's data, marketing individuals are doing this all over the place. They're able to take information that they know about you and predict other things that you're going to like, and target ads at you, right? So, it's like when you look up something on the internet and you say, I don't know, buy cat food, suddenly the next time you open your email and Google, there's ads for other cat food along the way because now they know, well, if you liked that cat food, you're probably going to like other things that we should know about.
Okay. So, there was sort of this key kind of public concern that Matthewx, in his article, How Cambridge Analytica's Facebook Targeting Model Really Worked, According to the Person Who Built It. And I guess the big thing that I want to point out here is that essentially what they're doing is they're taking all of these correlations and they're trying to soak it up together. So, it's not that they're making any inferences about you based on what you like and you don't like, it's that they're saying if you like this and hundreds of other people liked this other thing, were going to assume you like that too. They're not actually asking a question of why you like these things or why you feel the way they do. They're just going to soak all of this information up and be able to predict this along the way.
Okay. So when you're doing inference, this measurement error stuff really matters, right? It turns out that's kind of the key point of what I wanted you to understand. So whenever you're looking at test data, whether it be educational testing, whether it be tests within a medical environment or anything else, you really want to think about how much possible measurement error exists within that if you're going to use that to predict something else. But if all you really care about is getting predictions about particular individuals, you don't really much care about the measurement. What you really need is lots and lots and lots of data. Like Facebook, Netflix size, in order to end up with a good model.
I thought I would end by telling you that I was super excited when I took the Harry Potter quiz. I assume because I was a girl and I was nerdy, I would get Hermione. But it turns out I got Dumbledore, which kind of made me pretty excited along the way. All right. So, what questions do people have?